Drug discovery is a numbers game. Out of the millions upon millions of compounds that biopharmaceutical companies screen, perhaps only a handful will emerge as potential drug candidates.[1] This staggering attrition rate means companies need to screen compound libraries quickly and accurately.
Enter high-throughput screening (HTS)
HTS is defined as the screening of 10,000-100,000 compounds per day. Ultra HTS, or uHTS, is defined as 100,000+ compounds screened per day. These processes utilize orchestration robotics, liquid handlers, plate readers, informatics applications, and other various software with the goal of rapidly identifying compounds that modulate specific targets, pathways, or cells/organisms.
The reward for good work…
HTS currently dominates the early discovery landscape. Laboratories investigating small and large molecules, materials, and even cell-based therapies use HTS every day.
The technology is also improving. Since its widespread adoption in the 90s, the process has become increasingly efficient. Cost-per-tested entity has steadily declined as screening techniques have become more advanced, require less material per experiment, and employ improved technology.[2]
However, with the rise of ever more complicated modalities such as personalized therapies, biologics, and cell-based therapy, which deal with molecules 103-1013 times larger and more complex than classic small molecule compounds[3], the volume of compounds that need to be screened has outpaced HTS’s accession.
Keeping HTS effective in the era of big data
It may be comforting to know that even as screening technology improves, and the volume of investigational compounds sitting on deck trends toward infinity, the fundamental requirements for effective HTS remain the same.
The magic triangle of HTS

Time—HTS processes need to screen millions (and millions) of compounds every year. Each compound requires significant time for preparation and experiment execution. Additionally, these experiments generate massive amounts of data that need to be made available for the right people, quickly.
Quality—The information derived from each compound needs to be high quality. Both false positive and false negative errors increase the probability of advancing a poor drug candidate. Inefficient data handling practices like manual data processing or poor metadata attribution, increase the likelihood of these errors.
Costs—Each screening requires technology, data management, personnel, and active ingredients. Misalignment, bottlenecks, or inefficiency in any of these domains cause departmental stress and increase a laboratory’s price-per-well screened.
When companies struggle to uphold these fundamental principles there are significant costs, especially when considering the price associated with advancing poor candidates. A 2020 JAMA study found that advancing a successful drug (from preclinical through clinical testing) costs around $374 million. However, when accounting for failed clinical trials, the average cost per drug shoots up to $1.6 billion.[4] This means that, on average, over 75% of a biopharma’s drug development budget goes toward failed products. Catching (and discarding) even a fraction of these compounds with better early screening processes could save a company millions. But, achieving “the magic triangle of HTS” within the modern scientific data ecosystem has become a serious pain point for even the most advanced biopharmas.
The first glaring issue is how data is shuttled through workflows. Many HTS protocols still require manual data collection, curation, and processing to prepare data for informatics applications, structure-activity relationship (SAR) dashboards, or in silico modeling. These manual processes are slow and error prone. Furthermore, they force companies to pay Ph.D.-level employees to spend time uploading instrument data to file shares or PCs, adding metadata, and QCing data fields, all by hand. This may be a good time to re-emphasize that these workflows process millions and millions of compounds per year.
Traditional HTS workflow
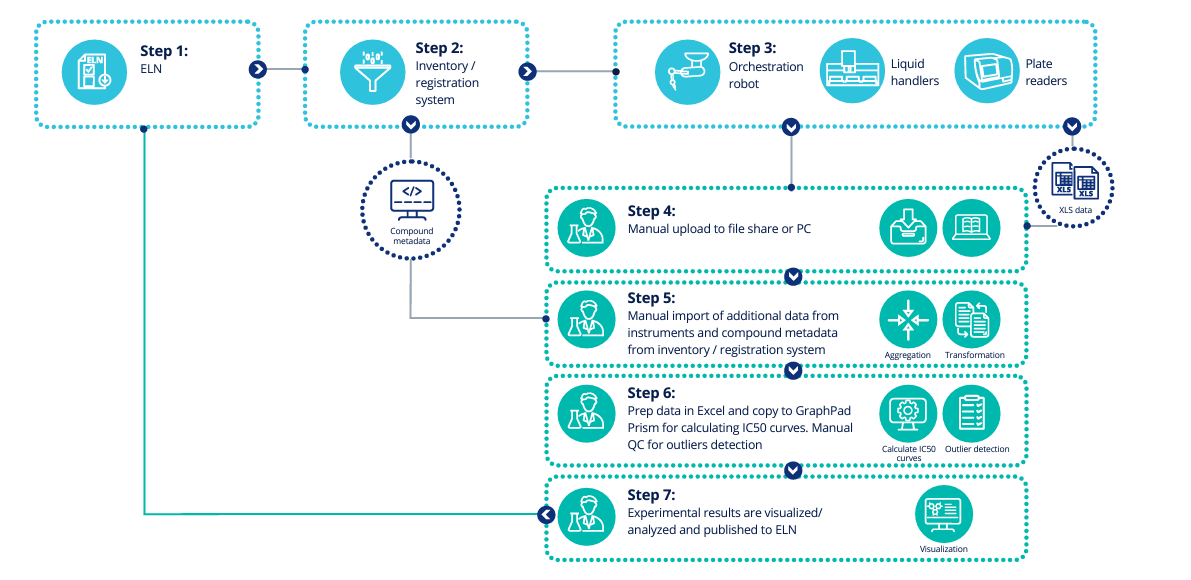
The second common issue is that companies lack a reliable way to manage and track what happens to data as it is processed. As a result, data records begin with raw data, enter a black box, and presto chango, processed data emerges. From a compliance or even a methodological perspective, this is untenable. Data without a provable history becomes useless and experiments have to be repeated.
The third concern is that poor data hygiene across HTS processes can lead to inaccessible, inoperable, unreliable, and lost data. This occurs when:
- Data emerges from instruments and applications in vendor-proprietary formats (read incompatible without processing)
- That data is then stored ad hoc wherever a team finds convenient (network shares, local shares, personal computers, wherever)
- Many teams either do not capture metadata or they capture metadata in inconsistent formats (one team says protein_test_1, the other team says pro.test.one)
This quagmire of disparate formats, local storage, network storage, and search-resistant data causes a knowledge hemorrhage. Scientific context is lost, search queries don’t function, SAR dashboards sit idly waiting for high-quality data, and in silico modeling becomes impossible.
I bought a spaceship. Now all it does is sit in the driveway
Biopharmaceutical companies are well aware that HTS process improvements hold tremendous value. Reducing implementation and support costs and providing better data (both access and quality) across their organization are often top priorities.
This is evidenced by their heavy investment in technologies surrounding HTS processes. Analytics software is improving hypothesis generation. This means fewer compounds can be screened without compromising the probability of success, reducing both material cost and labor. Advances in AI/ML-enabled computer-aided design, in silico libraries, and molecular docking software are also ways companies are trying to improve screening efficiency, increase predictability, and find compounds with clinical applicability faster.
However, despite the amazing power these technologies provide, they remain hamstrung by the concerns outlined above—manual data migration, lack of traceability, and poor data hygiene. Without a reliable, traceable, high-quality HTS data flow, there will continue to be a definitive ceiling over HTS efficiency, even with state-of-the-art modeling, analytics, and machinery.
Time to roll up our sleeves?
Some biopharma companies have started to triage their HTS issues with internal, DIY teams. What they quickly find, however, is that the time, effort, and cost to build and maintain scientific data workflows goes well past their initial expectations. Laboratories quickly fill with a patchwork of custom IT solutions that require intricate construction, thorough testing, and the elusive expertise of people who understand both HTS processes and scientific data engineering. The combination of these factors has created internal strife as time-to-value expectations exceed capabilities while screening demands continue to multiply.
Achieve your HTS goals with the Tetra Scientific Data and AI Cloud
With demands for HTS improvements piling up and in-house solutions struggling to produce value, biopharmaceutical companies find themselves searching for vendors who can help. That’s where TetraScience comes in.
At TetraScience, we are a unique blend of people who understand how data works and people who understand how biopharma laboratories work. We combined that experience to create the Tetra Scientific Data and AI Cloud.
The Tetra Scientific Data and AI Cloud is the only end-to-end scientific data solution that was purpose built to solve data problems in biopharmaceutical laboratories, including those associated with HTS.
Our technology and know-how help laboratories improve all three facets of HTS performance—time, cost, and quality—by addressing the major data-related issues facing HTS laboratories.
HTS workflow with the Tetra Scientific Data and AI Cloud


Bringing learnings forward
Just as HTS workflows have improved over the years, so have HTS data workflow solutions. TetraScience has stepped into some of the top labs in the world (14 out of the top 25 biopharmas trust us with their data) and created solutions that are delivering real world results today.
We have taken those lessons and created a pre-built solution that helps organizations get up and running with their scientific data quickly rather than spending months hiring personnel, building and testing a custom solution, and potentially never achieving ROI. With our productized offering, biopharmas receive best-practice solutions for their HTS workflows so they can start seeing better leads, faster, with a lower cost.
To learn more about how the Tetra Scientific Data and AI Cloud can accelerate and improve your HTS workflows, you can visit our website.
Are you a more tactile or visual learner? Request a demo today.
References:
[1]Aldewachi H, Al-Zidan RN, Conner MT, Salman MM. High-Throughput Screening Platforms in the Discovery of Novel Drugs and Neurodegenerative Diseases. Bioengineering. 2021. https://www.mdpi.com/2306-5354/8/2/30.
[2]Mayr LM, Fuerst P. The Future of High-Throughput Screening. Journal of Biomolecular Screening. 2008.
[3]AZBio. Small Molecules, Large Biologics, and the Biosimilar Debate. https://www.azbio.org/small-molecules-large-biologics-and-the-biosimilar-debate.
[4]Wouters OJ, McKee M, Luyten J. Estimated Research and Development Needed to Bring a New Medicine to Market, 2009-2018. JAMA. 2020.