With only 6.3 percent of drug candidates reaching the market, the average cost of their development has soared to over $2 billion. Clinical failures, primarily due to issues with efficacy (52%) and safety (24%), contribute largely to these costs. Consequently, the biopharma industry is keen to adopt "fail fast, fail cheap" strategies to weed out unfavorable candidates much earlier in development. Artificial intelligence (AI) is increasingly seen as the key to enabling these approaches.
Here, I outline a science-driven, data-centric approach that uses AI to evaluate properties of drug candidates related to their absorption, distribution, metabolism, excretion, and/or toxicity (ADME/Tox). Our AI model has significantly streamlined and improved the accuracy of in vitro testing. This work will pave the way for building a quantitative structure-activity relationship (QSAR) model that combines mechanistic insights from molecular structures and critical findings from ADME/Tox studies. Such a tool will empower scientists to evaluate drug safety earlier in the drug development process, resulting in faster timelines and lower costs.
Evaluating ADME/Tox with off-target drug panels
Off-target drug panels are critical to drug development. They test whether a drug interacts with unintended biological targets, usually proteins, within the body. These interactions may lead to adverse effects or toxicities.

In a typical assay, the activity of the off-target protein is measured with multiple dilutions of the drug candidate. This data is then used to generate a dose-response curve. A key metric calculated from this curve is the half-maximal inhibitory concentration (IC50), that is, the concentration of a drug needed to decrease activity by 50 percent. Lower IC50 values indicate a higher likelihood of adverse effects.

Increasing the number of sampling points can improve the accuracy of IC50 calculations. The tradeoff, however, is added cost and time. AI offers a promising alternative. By modeling the interactions between drugs and off-target proteins in silico, we can improve IC50 estimates using fewer data points. In other words, we can use AI to minimize the need for new experimental data by leveraging historical datasets and existing knowledge.
Exploratory data analysis and model training
To develop an AI model for ADME/Tox prediction, we undertook a four-step process:
- Leverage knowledge graphs to constrain the data. Knowledge graphs provide a framework for understanding the relationships between various entities within a dataset. This structure aids in filtering out irrelevant data and emphasizing information that is pertinent to specific queries or tasks.
- Apply scientific knowledge to hypothesize causal mechanisms. This process combines publicly available domain knowledge with sophisticated data analysis tools to rigorously and systematically uncover true causal relationships between variables. This ensures that our findings are not only based on coincidental correlations but are in fact indicative of real effects.
- Replatform and engineer data, followed by feature extraction. AI models require large-scale, liquid, and purpose-engineered datasets. We compiled and replatformed a diverse array of data—including molecular descriptors, assay conditions, and historical experiments and reports—using the Tetra Scientific Data and AI Cloud™. The data was then transformed into an open, vendor-agnostic format and contextualized with scientifically relevant metadata. We then conducted feature extraction on this AI-native Tetra Data to reduce data complexity while preserving relevant information.
- Use historical and new experimental datasets to train the machine learning (ML) model.

Optimizing off-target assays with AI
We first used the ML model to improve how scientists perform off-target panels, specifically drug transporter assays, to calculate IC50. The model recommends which drug concentrations to test in an experiment. It leverages information from historical data as well as previous sampling points to predict the next optimal concentration.

In a pilot study, this novel approach cut the number of sampling points in half while improving the accuracy of IC50 calculations. Conventional curve-fitting strategies are more susceptible to experimental noise, resulting in higher errors.

Next steps: QSAR
After a successful pilot, we are currently validating our method. In the next phase of the project, we plan to develop a QSAR model that builds on our earlier efforts. The goal is to predict ADME/Tox properties of compounds in silico based on their molecular structures. This enables scientists to screen compounds for safety at an early stage in discovery. By doing so, they can minimize downstream testing and substantially reduce overall costs.
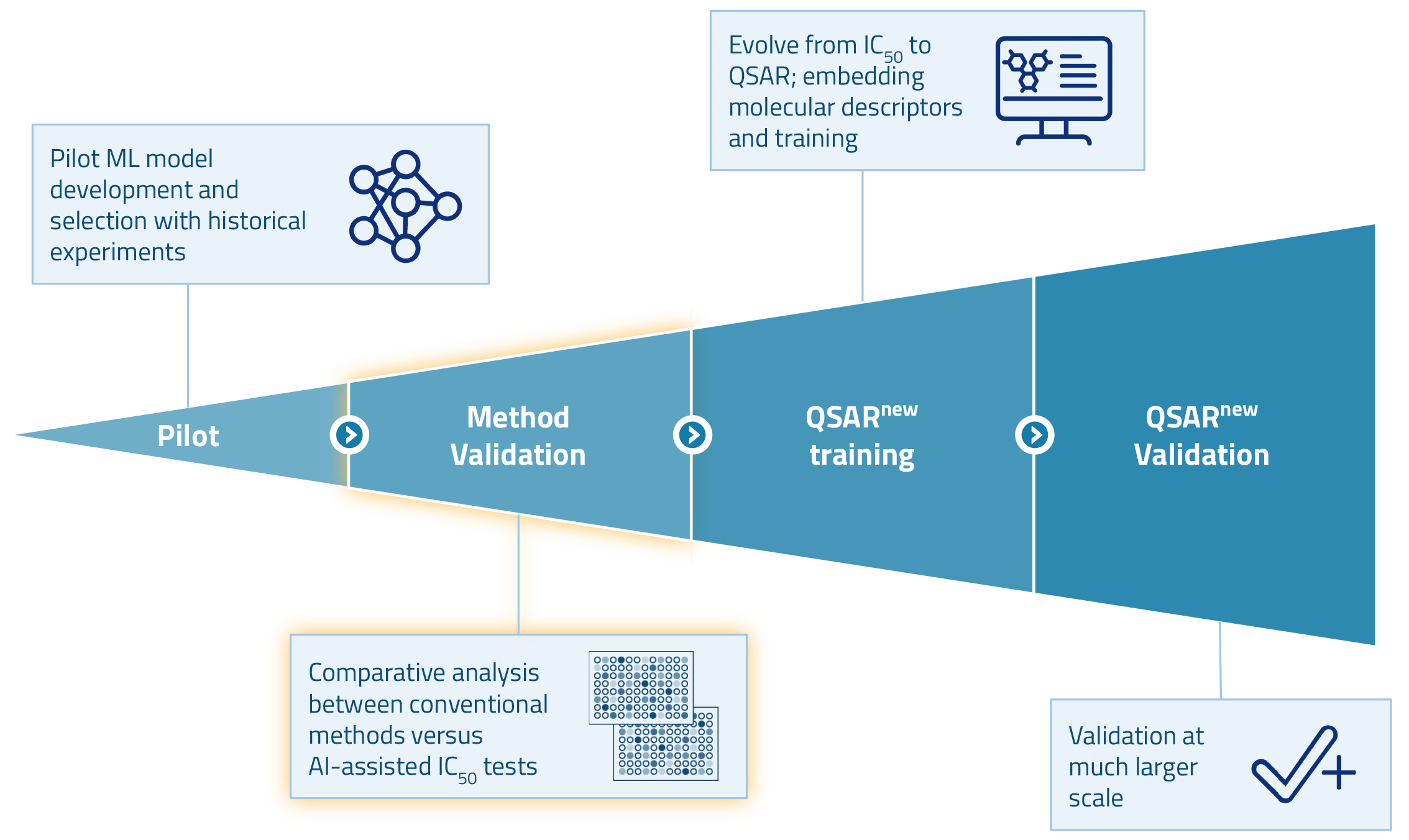
Interested in learning more? Read our case study on AI-assisted ADME/Tox testing.