We have gained many insights since TetraScience first started to tackle challenges related to scientific data in the biopharmaceutical industry. There has been a tremendous evolution in how scientific data is generated, moved, analyzed, and stored. These are macro-trends that go far beyond a single company. Despite this, we are only at the beginning of a radical transformation that is underway in scientific data management and analysis.
Scientific data
To understand the reasons for this shift, we must first examine the industry’s core asset—scientific data. It is dispersed throughout a biopharma organization, generated by many groups in many locations. For example, consider a protein purification group and all the instruments they use, spread across various labs, floors, buildings, and sites around the world.

When taking into account all scientific groups within an organization—including those working on high-throughput screening, characterization, quality, and many other activities—there are collectively thousands of places where files are stored, all locked up in data silos. This fragmentation makes scientific data incredibly complex and poses major challenges.

Paradigm shift
Companies around the world are waking up to a realization: they have been mishandling and underutilizing their scientific data assets. Increasingly, they are moving beyond traditional laboratory connectivity and outdated data management strategies to establish a foundation for analytics and Scientific AI. As a result, there is a paradigm shift underway in scientific data management. It’s catalyzed by the need to effectively analyze scientific data while leveraging analytics and AI. It’s independent of any individual company. And it’s only just beginning. Let’s examine the many benefits of this new paradigm.
From on-premises to cloud-native data management
The transition from on-premises, siloed infrastructures to cloud-native services has revolutionized data management. A cloud-native solution offers a lower total cost of ownership, better scalability, and support for the high workloads that advanced analytics, machine learning, and AI demand. This shift is propelled by advancements in AI and machine learning by leading tech companies, further enhancing the capabilities of scientific data analysis.
From file-based to engineered data
Next, there is a transition from file-based data to engineered data that's readily usable by AI and analytics tools. Files segment your data in containers that limit access. Scientists are not interested in the file per se but rather the information it contains. Purpose-engineered data includes critical scientific context, uses multimodal taxonomies and ontologies, and can provide insights for data reuse with any scientific application.
From in-line data processing to event-driven processing
There is a growing recognition that simple point-to-point integrations are inadequate. In many of these cases, data management is tied to an instrument or application suite. The workflow is linear, consisting of storing, then processing data, and exploring use cases in an inefficient, iterative way. For new use cases, it can be difficult to extract useful data, as it often needs reformatting. An event-driven data processing model using tools provides efficiency gains through flexible, customizable, on-demand data processing and engineering that can branch and evolve with your use cases—all with built-in data versioning and lineage.
From disjointed analysis to co-located cloud-based data apps
Next is a shift from data manually moved between laboratory instruments and analysis software, to data that is co-located with applications in a cloud-based data workspace. Typical analysis workflows are manual-intensive and involve multiple data stores, making processes slow and error-prone. It is preferable to have all of the data in one single place, from where scientists can easily retrieve data, upload it into their preferred tools, and share their work across project stakeholders. We learned that bringing analytical software into the cloud, where the data is co-located, will result in seamless access to data, reduced errors, better collaboration, and increased scientific productivity.
From endpoint-specific SDMS to endpoint-agnostic data cloud
Another element is the move from endpoint- and vendor-specific stores to a data-centric and endpoint-agnostic data cloud. Many endpoint-specific instrument vendors offer SDMS as part of their suite, which creates a heterogeneous landscape of vendor-walled gardens. As a result, there is a growing trend to adopt horizontal cloud solutions that are detached from the endpoint. This provides independence from vendors and enables large-scale, liquid, and FAIR datasets, which provide the foundation for AI and advanced analytics applications. To ensure the data cloud does not become another silo, it must be part of a data mesh, where data can be consumed irrespective of its source.
From single-company to multi-company collaboration
Five years ago, industry conversations focused on "my company’s data." Since then, outsourcing has been taken to the next level. Data management strategies must now be designed to support data sharing between companies due to the prevalence of external collaboration. Organizational boundaries, including security, data ontologies, and cadence, can restrict the flow of data, reducing it to a mere trickle of critical information that limits deeper collaborative insights. The new paradigm will create a substrate for collaboration across an ecosystem of partners where liquid data with common ontologies can be securely shared.
From IT project to data product
Data integration projects often prioritize technology over results. When irrigating a field, the value lies not in the pipes but in the crops. So, too, should moving data be less about the technological “plumbing” and more about the scientific use cases that emerge. The better approach is to focus on the data product. By including scientific stakeholders early on in the planning phase as well as in the execution, product owners will be more in tune with desired outcomes. Plus, they can unlock new scientific use cases by focusing on scientific data instead of IT technology.
From file management as an end state to an onramp to analytics, ML, and AI
Scientific data management does not have to be an end state for your data anymore. Instead of focusing on how to consolidate all of your data, the emphasis should be on what you want to achieve with it. How will the data be used today? What other insights might you need tomorrow? Scientific data management is a starting point, with a scientific data and AI cloud serving as the foundation and onramp to analytics and AI.

TetraScience prepares data for consumption
The Tetra Scientific Data and AI Cloud™ provides you with a data foundation for your analytics and AI while fulfilling your immediate connectivity needs. We provide core software to cover layers 1 and 2 (see below) right out of the box. These layers serve as the foundation for analytics tools, including those found in the Tetra Data and AI Workspace like FlowJo.
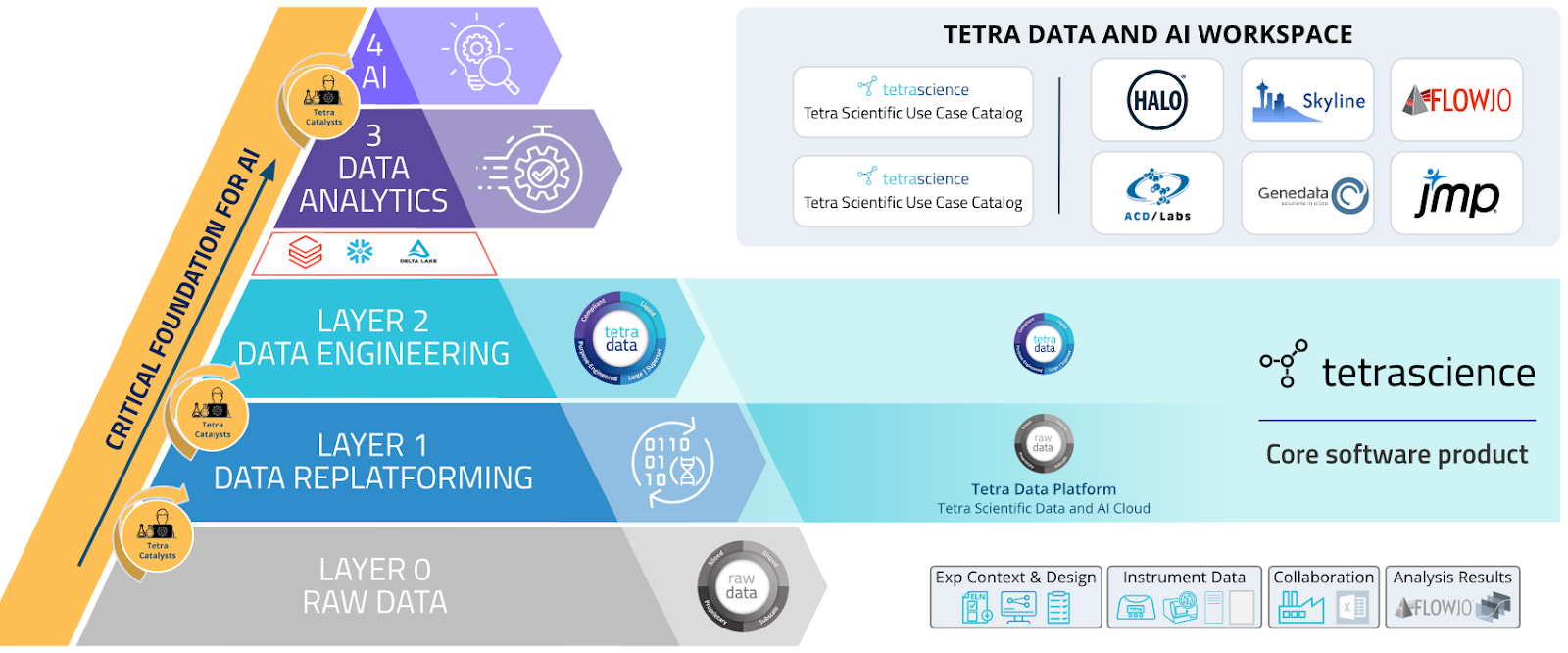
Conclusion
Under this new paradigm, the future is bright for scientific data management. Data will no longer be relegated to stagnant storage endpoints. It will be transformed into insights that propel innovation through advanced analytics and AI.