Genius of “And” – You can get both
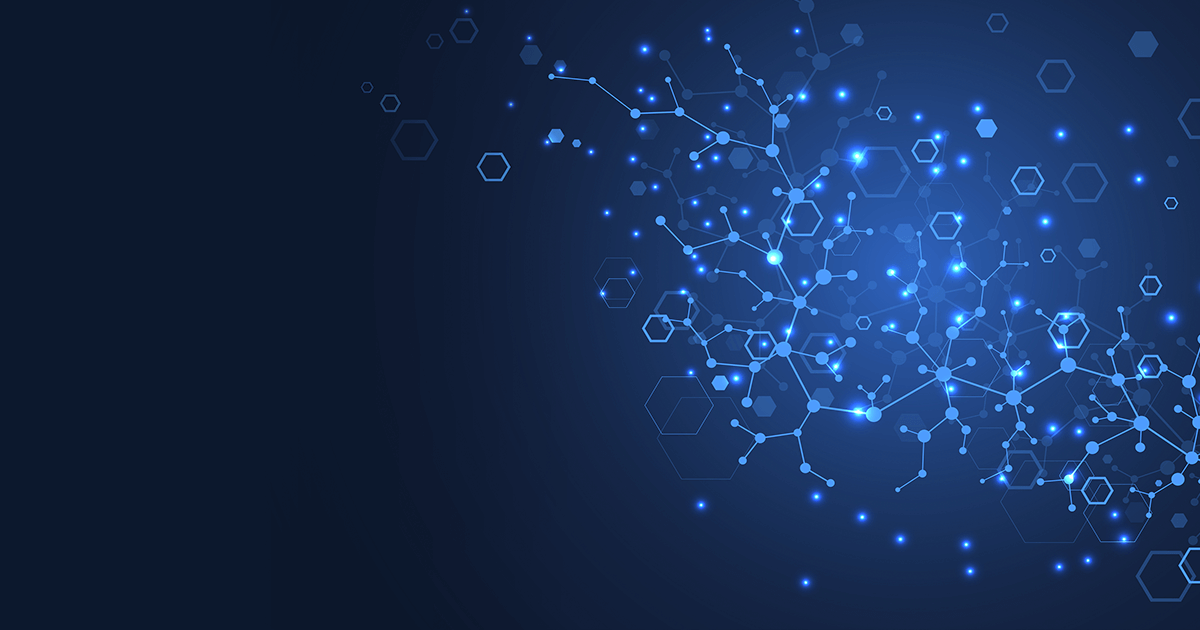
One common question we have seen from biopharma companies is that customers who would like to connect lab instrument software to an Electronic Lab Notebook (ELN) or Laboratory Information Management System (LIMS) are asking why they would need a Scientific Data and AI Cloud? Isn’t a middleware system sufficient? Why would they need to engineer data or get support by experts with hybrid expertise in both science and technology (we call them Sciborgs)?
We want to elaborate our fundamental thinking and explain the foundational difference between a Scientific Data and AI Cloud vs. a middleware approach.
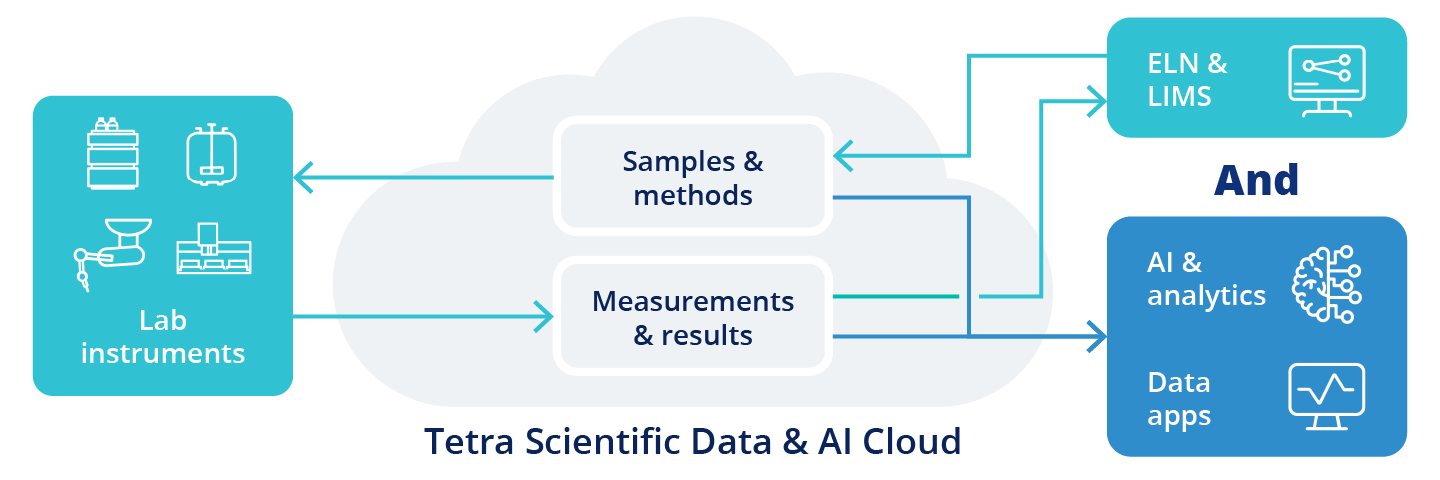
The middleware approach
Let’s take the example of a QC lab with a chromatography data system (CDS) where they would like to avoid the tedious, laborious and error prone manual process of entering peak values into LIMS. Therefore they are seeking a data automation solution to automatically get data collected from the CDS and enter it into the LIMS. They may choose to lean on middleware, purpose-built software that acts as an intermediary layer to transfer data between instruments and applications.
In this case the middleware approach will only extract some very limited information, such as peak area and height and analyte name, since these are the only fields needed by a LIMS report or an ELN schema. This has been the dominant approach, adopted by traditional LIMS vendors and system integrators.
The question we get is “why should we extract all the scientific data and engineer the data when I only need several numbers to be entered into my LIMS and choosing a middleware is enough?”
The reason is that this approach has one major flaw since it extracts data based on what the LIMS needs for result generation or what is defined in the LIMS report. We call this approach “middleware approach” or “single endpoint-driven approach”. LIMS and ELN are designed and used to track workflow, samples and batches, record experiments, and produce specific reports. These are applications tailored to the scientific workflow, thus not meant to hold all the data.
The middleware approach is entirely designed for connectivity with one endpoint and the middleware serves what that endpoint needs, thus presenting a terminal dead end for scientific data without the potential of unlocking its value for analytics and AI. The approach is not data centric, it is endpoint or application centric. Because of this, significant rework of the connectivity solution is needed when organizations need to reuse the data for other applications or leverage analytics and AI to derive scientific insights and improve and accelerate scientific outcomes.
In the last several decades, the middleware approach has been the dominant pattern adopted by the biopharma industry, heavily influenced and perpetuated by industry dynamics that are application centric and instrument centric:
- LIMS/ELN vendors are reliant on system integration service revenue and try to lock as much data into their application suite as possible
- The handful of traditional scientific data management systems (SDMS) are all provided by instrument manufacturers.
Genius of “And” with the Tetra Scientific Data and AI Cloud
In contrast to this, TetraScience takes a “data-centric/first” approach, that extracts all the scientifically relevant data from the data source. In the case of chromatographic data, TetraScience extracts and engineers the following:
- Results, peak
- Mass spectra
- Chromatograms
- Method
- System (including chromatography columns)
- Users
- Samples
- Audit trail and events
This is a core design approach in the “intermediate data schema” strategy (read more here). This data-centric approach allows TetraScience to extract and engineer comprehensive scientific data, such that the engineered dataset can enable multiple scientific use cases, be leveraged by multiple applications (including ELN/LIMS), and especially analytics and AI.
The reason we chose the scientific data and AI cloud approach is because of our conviction that AI and advanced analytics will truly introduce step-function changes to biopharma organizations in terms of discovering, developing and manufacturing drugs faster, cheaper and safer. But this is only possible through purposeful engineering of large-scale data for science.
In order for biopharma companies to leverage their scientific data for advanced analytics and AI, the scientific data must go through an immutable order of operation as illustrated in the following graphic.
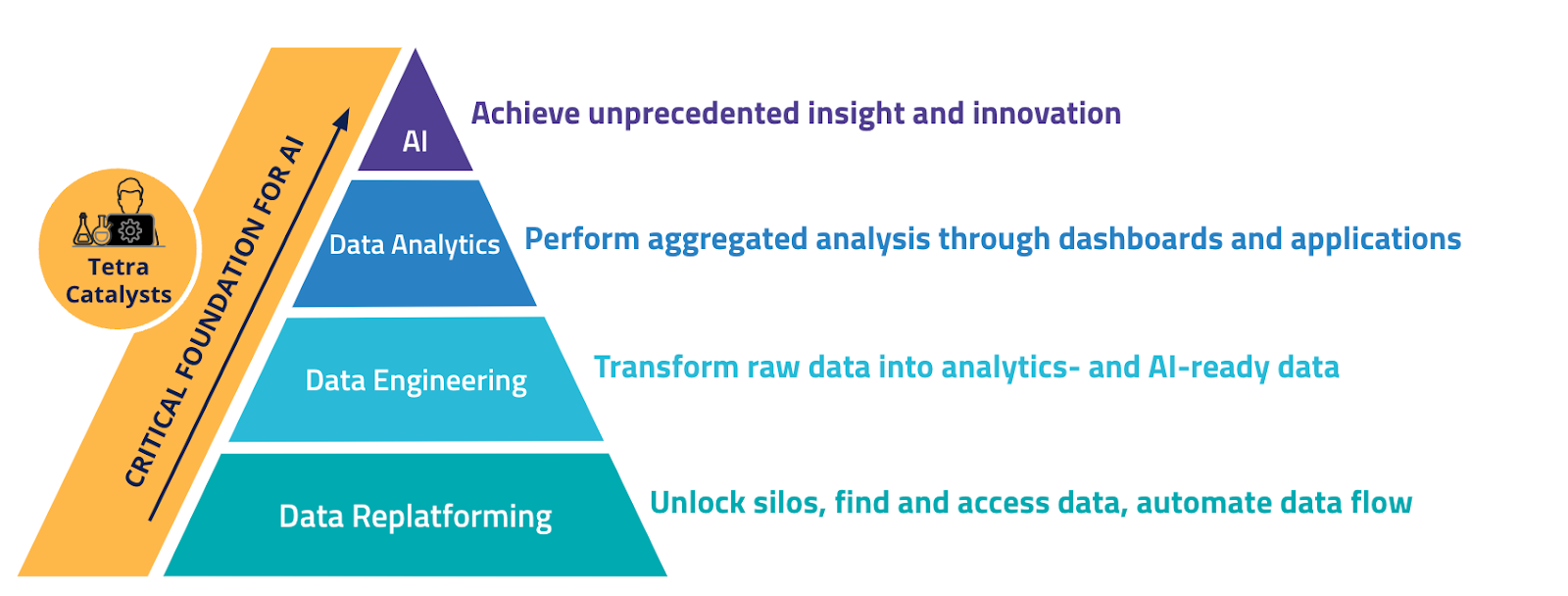
Lab connectivity projects that are automating the entry of data into ELN/LIMS are common use cases for data replatforming (layer 1). But this is not enough. The scientific data and AI cloud approach treats lab connectivity as one of the initial milestones to get to the top of the pyramid. In order to accomplish a company’s vision to leverage AI and analytics, connectivity is only the first step. It is required to unlock the data from thousands of siloes, but replatforming comprehensive scientific data must be followed by engineering this data to be analytics- and AI-ready. Only then can customers achieve high-impact innovation by addressing immediate connectivity needs while also transforming their scientific data for analytics and AI- the genius of “And”.
With the Tetra Scientific Data and AI Cloud, connectivity is achieved using a data-centric manner – data is replatformed and engineered to preserve all scientifically meaningful information, then the data can be published into the ELN to populate a notebook entry, can be published to another application, and can be used for analytics and AI. The Tetra Scientific Data and AI Cloud uses analytics and AI as forcing functions for data replatform and lab connectivity. It is designed to enable both “lab connectivity” use cases and sets a data foundation for analytics and AI.
Tetra Catalysts and Sciborgs
Connectivity is an important piece of the data journey and needs to incorporate elements of discovery and collaboration with scientists. Middleware developers may lack the nuanced understanding of scientific context, value and scope of use cases, which can result in a product with an unsustainable foundation and niche utility. In contrast, the Tetra Scientific Data and AI Cloud approach is supported by embedded experts with a hybrid skill set of science and technology known as “Sciborgs”. They are able to perform use case discovery, design processes and data workflows in deep collaboration with scientists that drive business outcomes and adoption, as well as provide sustainable support for scientists and promote the reusability of the data for analytics and AI.
Summary: the middleware approach is a terminal dead end, the combination of lab connectivity and a data foundation are the future for analytics / AI
A middleware approach is entirely designed for connectivity with one endpoint, thus representing a terminal dead end for scientific data to move up the pyramid to unlock exponential value. It is the opposite of “data centric”, it is application centric, resulting from a single endpoint.
Because of this, significant rework of the connectivity solution is needed when organizations with a middleware approach need to reuse the data for other applications or strive to leverage analytics and AI.
With the rise of applying data analytics and AI to achieve step-function changes in the biopharma industry, you need to do both, solve immediate connectivity needs and build a foundation for analytics/AI. Only a data-centric approach that is the basis of a solution like the Tetra Scientific Data and AI Cloud can fulfill these requirements and allow customers to harness their scientific data to improve and accelerate scientific outcomes.
Ready to discuss your use case? Talk to a TetraScience expert.