Automated Anomaly Detection and Correction
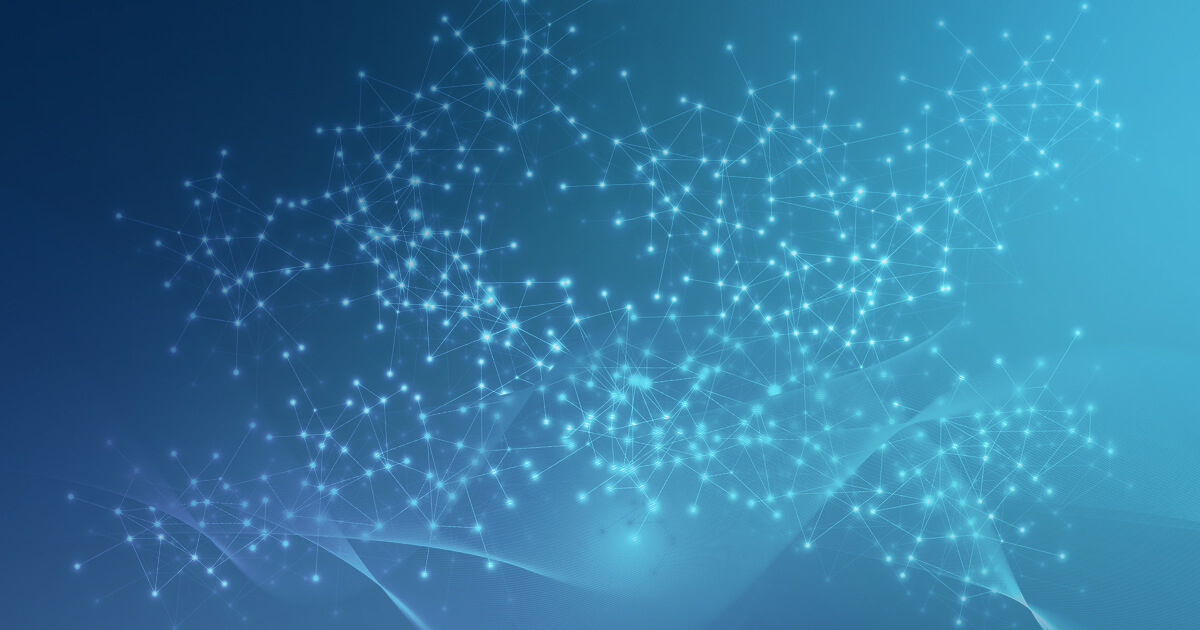
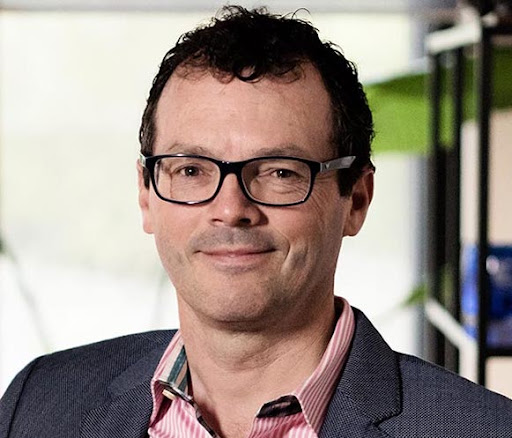
Randall Julian is CEO, president, and founder of Indigo BioAutomation (originally, Indigo Biosystems) located in Indiana. Randy earned a PhD in chemistry from Purdue University in 1993 and then worked in discovery chemistry at Eli Lilly for 14 years. He founded Indigo based on informatics technology developed in his research group and has led Indigo from its founding to profitability, building a world class management, engineering, and research team to commercialize laboratory data analysis software.
Dr. Julian is a frequent speaker in the mass spectrometry community and teaches short courses in statistics and data analysis. Randall is the past chairman of the Human Proteome Organization’s Proteomics Standards Initiative steering group where he co-authored two international standards for analytical data. He was also the chairman of the ASTM committee on analytical data standards. Dr. Julian also maintains an active research relationship with the faculty at Purdue University where he is an adjunct professor of chemistry.
We recently talked with Randy to find out more about Indigo and the value of the Tetra Partner Network. We’re also hosting a webinar with Randy. Please register for AI/ML Based Anomaly Detection for Improved Scientific and Regulatory Outcomes.
Randall, before we dive into what Indigo accomplishes today, please tell us a bit about your background and why you founded the company.
While working on my analytical chemistry PhD in the early 90s, I noticed people trying to do computational research on new instrumentation using early PCs. Back then the difference in power between a PC and a high-performance computing system (HPC) was huge. Using a PC limited the scope of what could be done so I ended up using the Cray supercomputers at Purdue, and the Connection Machine at Los Alamos National Laboratories to build models that could be used to build new instruments.
It was the analytical chemistry equivalent of designing an airplane with a computer model rather than with a calculator. It opened my eyes to how little impact our best computers had in chemistry outside of a few physical chemistry problems. At Eli Lilly, my team applied advanced hardware and software to analyze instrumental data to speed up natural products drug discovery. Those projects eventually grew into our present company, Indigo BioAutomation.
Indigo uses advanced algorithms and high-performance computing systems (now based in the cloud) to automatically analyze complex data from clinical and life science laboratories. Our products use models of the physics and chemistry of instrumentation to identify problems with instrument performance and individual samples that are not easy to detect with the human eye. Since we are not limited in computational power or storage, we've developed some very powerful, and explainable AI/machine learning (ML) solutions for high throughput clinical diagnostic tests and drug development processes.
For example, we accepted a customer challenge to help improve turn-around time for COVID-19 PCR testing. Since Indigo's flagship product, ASCENT, successfully uses a mathematical model of liquid chromatography and mass spectrometry (LCMS), we built a model that represents the PCR reaction. Within about a week, we were able to process PCR data with the same degree of automation as we are for LCMS. ASCENT processes about 40 million samples a year with no limit in sight. Now, the PCR product, called ARQ, has the same capability. In addition, ARQ has reduced result review time by 75 percent in one infectious disease laboratory.
We have also developed and delivered a mathematical model for the signals generated by a new multiple myeloma test. Again, using models of chemistry and physics, combined with advances in ways to use models, we believe the new system can detect cancer at sensitivity levels thousands of times better than what is possible today.
In clinical laboratory testing, everything is urgent. A poor laboratory result will affect people that day. How does Indigo help clinical labs and how can your experience with clinical transfer to biopharma research?
We have all learned from the COVID-19 pandemic the value of fast, reliable test results. The need has always been there, but now vast numbers of people are being directly affected by testing. Historically, if you were automating something, the focus was on cost savings which automatically makes people think of eliminating jobs. That’s not really the case in today’s labor market. It’s more important to help labs use their limited staff to deliver on unprecedented volume spikes.
Automation makes tests we all need faster, better, and cheaper while making the lives of people in the lab better by shifting tedious, repetitive work to machines. Automation in health-related laboratories frees people to do what humans do best: work in teams to accomplish what no one person can do by themselves. That requires critical thinking, problem-solving abilities, and above all, the ability to communicate and collaborate across the lab with other experts.
Automation also allows for data-driven improvement programs to solve problems in the lab that affect everything from the quality of the results to the quality of life in the lab. By having data that can be analyzed for overall system performance, labs can continuously improve in all the dimensions that are important to them.

It seems like you understood early in your career how important computational science and technology would be to advancing healthcare. Has there been any downside to the digital revolution?
Analytical measurements are critical to every aspect of the life sciences, from basic research to primary health care. The proliferation of computers has led to significant advances in measurement technology and automation. Since then, we have moved away from dependence on paper recordings of measurements and documentation of everything from standard operating procedures to data analysis and summarization in paper notebooks. While not complete (the use of sticky notes at the bench is alive and well), the shift away from paper-only records is a good thing.
"The digital revolution, however, is decidedly doubled-edged....We are now at a point where it has become challenging to tell what happened during an experiment or what an observation means."
Digital analysis of digital data is far superior to hand analysis with calculators. Recording results in digital files, storing them in databases accessed by electronic notebooks or laboratory information systems, and using powerful algorithms for data analysis has revolutionized all aspects of healthcare. The digital revolution, however, is decidedly double-edged
Given the complexity of biology, it was inevitable that this complexity would show up in the diversity of data, methods of analysis, and types of records we keep while working on biological systems. Data storage, computing power, and measurement complexity all grew to match the complexity of our questions. The more difficult the problem, the more complicated the disease, then the more complex the chain of events leading to any conclusion will be.
We are now at a point where it has become challenging to tell what happened during an experiment or what an observation means. Experiments and measurements are so computerized that, ironically, it has become tough for scientists to record all the context needed for anyone to understand what they have done.
The stakes are high:
- Diagnostic laboratories are detecting terrible diseases.
- Drug companies are designing critical treatments and preventions.
- Healthcare professionals work overtime to provide patients with the best care and advice.
- Regulators are working to ensure the quality of diagnosis, treatment, and care, because lives are on the line.
Let me illustrate the situation using the measurement of the quantity of a compound in a complex mixture as an example. In the diagnostic setting, this could be a marker of the recurrence of cancer measured in blood. In drug discovery, it could be the drug levels in the body measured over time. In manufacturing, it could be the presence of a contaminate or side reaction product.
All these measurements are critical to the delivery of healthcare today. All are done with complex instruments with sophisticated quality control processes and done under the oversight of internal and external regulatory groups who represent society's interest in getting the results right.
The situation is made much worse when many groups in different locations are part of a team performing this work. With centralized data aggregation and automated anomaly detection, the basic processes of clinical diagnostics and drug discovery and development can be streamlined.
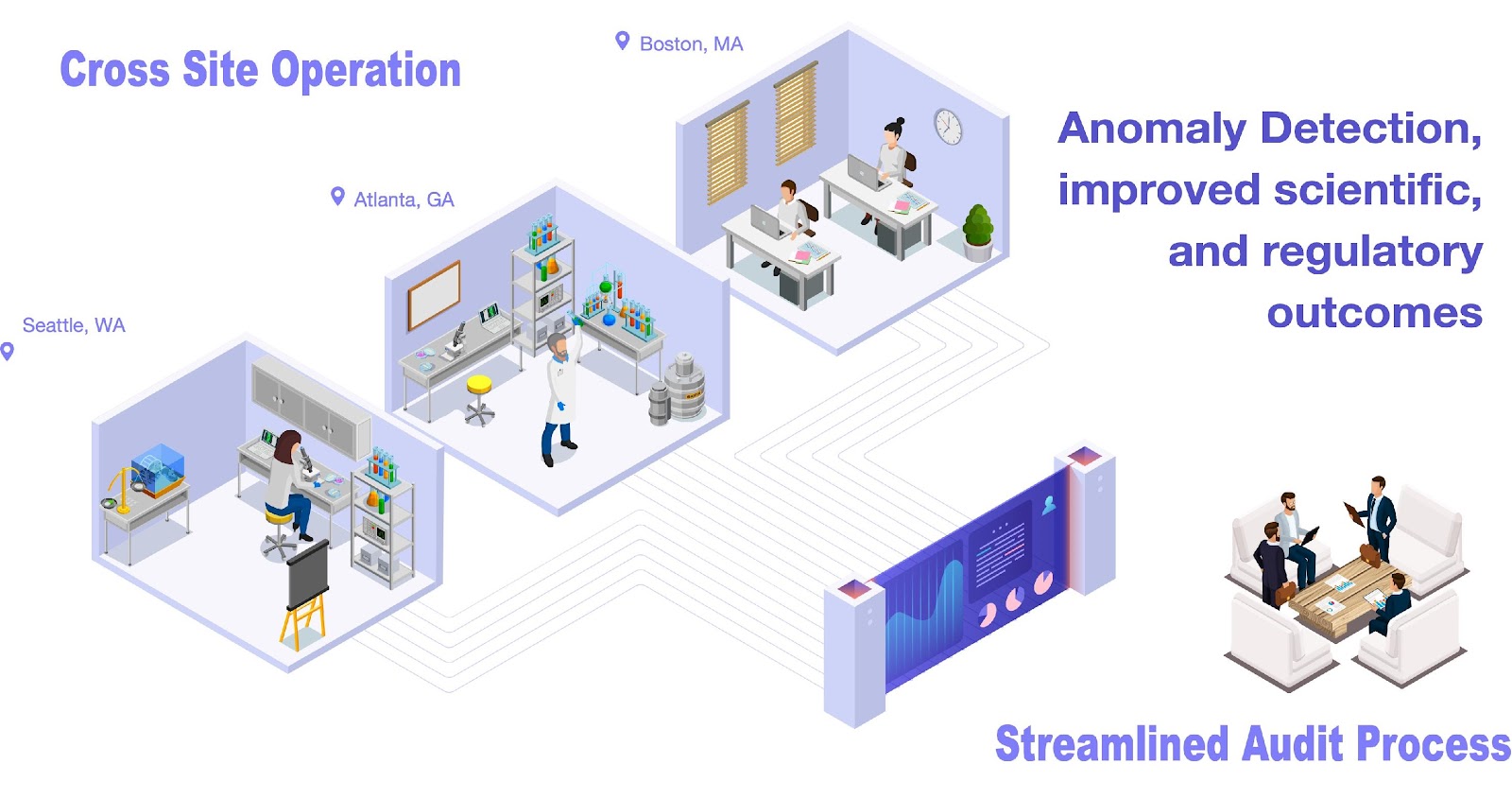
How do Indigo’s products alleviate the burden of decision making from the scientist?
The difficulties I mentioned have been known for quite some time. They have been the subject of tremendous work by dedicated measurement scientists, computer scientists, and experts on regulation and the use of evidence in law.
The first necessary condition for understanding what happened during a measurement is to organize a wide range of data types in a highly trusted and easily usable system. The data must include information about all the devices involved, the actions of people, and the timing of everything.
The second requirement is automatically finding anomalies in this highly diverse data. In real-world settings, anomaly detection is incredibly difficult for the simple reason that anomalies are rare events. That means that having deep expertise in detecting weak signals of trouble in the presence of normal variation (noise) is essential.
Indigo BioAutomation has been performing this type of signal analysis and anomaly detection for clinical diagnostic laboratories for over a decade. One of the elements of a successful system comes from machine learning: converting raw data into features that describe behaviors in a laboratory setting and using them to classify events.
"One of the elements of a successful system comes from machine learning: converting raw data into features that describe behaviors in a laboratory setting and using them to classify events."
A system can use standard AI techniques to evaluate data against an SOP to determine data reliability. But the input into such a system requires extracting relevant features from sources like text audit logs, timestamps of calibration events on balances, instrument error codes, measurement variations on QC samples, robotic system message logs, and even human actions.
Statistical analysis of historical data using machine learning algorithms is helpful for some features. In contrast, others require natural language processing of electronic lab notebooks, audit trails, and system logs. No single approach will detect rarely occurring problems in a laboratory or manufacturing process.
How can anomaly detection improve the drug development process?
Once an anomaly is detected, the most important thing is to prevent the unexpected situation from causing harm. Actions might need to be immediate, like shutting down a system. Or the problem may be best handled by alerting the appropriate people so they can decide what to do. Hopefully, simply connecting the right people to the right data at the right time can prevent a cascade of damage and cost because the later a problem is found and addressed usually, the more damage and cost it inflicts.
We now have the tools needed to allow a complex system to monitor itself. First-order automation enables computers to control devices. Second-order automation monitors an entire system. Our new technology gives everyone from the bench technician to scientists to regulators confidence that when we match the complexity of our tools to the complexity of the problems we are trying to solve, we can still trust the answers and move forward with confidence.
How does being a part of the Tetra Partner Network solve some of the problems you’ve outlined?
Detecting anomalies in any life science laboratory or process is problematic because it requires capturing enough context to detect rare events and distinguish them from noise. By partnering with TetraScience, Indigo’s algorithms now have access to a much richer data collection allowing even more precision and accuracy in detecting problems. Further with the scope of the Tetra R&D cloud, partnering with Tetra broadens the types of issues that we can pick up. Indigo can now support scientists through every phase of their work by automatically checking to ensure the results they record are supported.
What’s the value to customers?
Regardless of where a person works in healthcare, they want their results, decisions, treatments, and processes to be correct. If work isn't done right, someone could get hurt. As a result, there are harsh consequences for bad work or for not being able to explain or reproduce good work credibly. By catching problems early, the immense and painful costs in time, energy, and money to correct them later are avoidable.
With so much focus on the safety and efficacy of pharmaceuticals, it is critical that companies can answer all the questions along the drug development and manufacturing path before they slow a new drug submission when patients are waiting. Since it can take years to get a drug from development to the approval stages, everything needs to be in order when the work is done, not recreated for submission. Finally, if deviations are corrected and documented in real-time, internal and external auditors will have confidence that all regulated work is being done according to the required procedures. The benefit to the customer can be years of additional revenue from getting to market faster. The benefit to the patient can be years of their life.
"The benefit to the customer can be years of additional revenue from getting to market faster. The benefit to the patient can be years of their life."
Register for the Indigo BioAutomation and TetraScience webinar: AI/ML Based Anomaly Detection for Improved Scientific and Regulatory Outcomes.